The Big Rock Credit Union analyzed employee data to determine the factors that influence absenteeism. A regression model used the seasons, transport expense, distance from work, age, education, weight, height, and BMI to determine how these factors affect absenteeism
Below is the output of the regression analysis
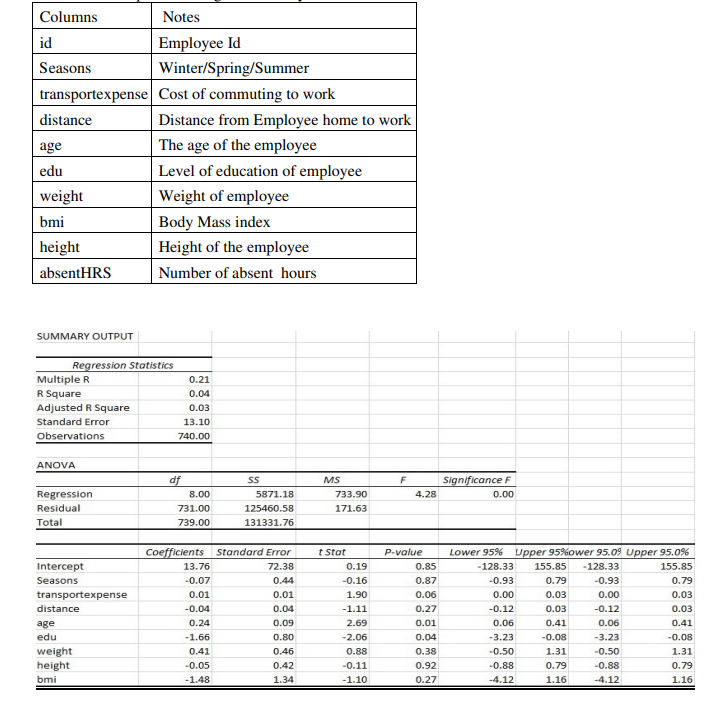
a. Explain the output. Include the R-Square and P-value in your explanation
b. Use the output to create a regression model for employee absenteeism. In your model include only factors that are significant predictors of absenteeism
c. Based on the model which factors should management focus on to address the absenteeism problem
\begin{tabular}{|l|l|} \hline Columns & Notes \\ \hline id & Employee Id \\ \hline Seasons & Winter/Spring/Summer \\ \hline transportexpense & Cost of commuting to work \\ \hline distance & Distance from Employee home to work \\ \hline age & The age of the employee \\ \hline edu & Level of education of employee \\ \hline weight & Weight of employee \\ \hline bmi & Body Mass index \\ \hline height & Height of the employee \\ \hline absentHRS & Number of absent hours \\ \hline \end{tabular} SUMMARY OUTPUT \begin{tabular}{lr} \hline \multicolumn{2}{c}{ Regression Statistics } \\ \hline Multiple R & 0.21 \\ \hline R Square & 0.04 \\ \hline Adjusted R Square & 0.03 \\ \hline Standard Error & 13.10 \\ \hline Observations & 740.00 \\ \hline \end{tabular} ANOVA \begin{tabular}{l|rrrr|r} \hline & df & \multicolumn{1}{l}{ SS } & MS & \multicolumn{1}{l}{F} & Significance F \\ \hline Regression & 8.00 & 5871.18 & 733.90 & 4.28 & 0.00 \\ \hline Residual & 731.00 & 125460.58 & 171.63 & & \\ \hline Total & 739.00 & 131331.76 & & & \\ \hline \end{tabular}